ChatGPT is a large language model that has been trained on a dataset of conversational text. It is able to generate human-like responses to text-based prompts and can be used for a variety of natural language processing tasks, such as language translation, text summarization, and question answering. The model was trained by OpenAI.
Google and all tech giants are now heavily investing in Artificial Intelligence (AI) due to its potential to revolutionize the way we interact with technology. AI can be used to automate tasks, improve customer experience, and provide insights into user behavior.
AI is being used in many industries such as healthcare, finance, and retail. Google has been using AI for years now in their search engine algorithms and other products such as Google Assistant.
Other tech giants like Amazon, Microsoft, Apple have also invested heavily into AI research and development. With the increasing use of AI by these tech giants, it is likely that AI will become an integral part of our lives in the near future.
Quick Links
What is Chat GPT
Chat GPT is a large language model developed by OpenAI, a research laboratory consisting of the for-profit OpenAI LP and its parent company, the non-profit OpenAI Inc. The project was first introduced in November 2022 by OpenAI, with the aim of creating a powerful language model that can understand and generate human language.
The model is based on the GPT (Generative Pre-training Transformer) architecture and is fine-tuned on a massive dataset of text to perform various Natural Language Processing (NLP) tasks such as language modeling, text generation, and text completion.
The main aim of the Chat GPT project is to create a powerful language model that can be used to improve natural language processing and generate high-quality, engaging content. The model is designed to be highly versatile and can be fine-tuned for a wide range of applications, including content creation, question-answering, and conversation generation.
The project is aimed to advance the state-of-the-art in natural language processing and machine learning and to provide researchers, developers, and businesses with a powerful tool for creating intelligent applications and services
How to Create an Account on Chat GPT
There are very few simple steps to create an account to Chat GPT:
1)- Click on the Chat GPT sign-up page at https://chat.openai.com/auth/login
2)- Enter a valid email address and put in a password
3)- Verify email and contact number
4)- Login and start using Chat GPT
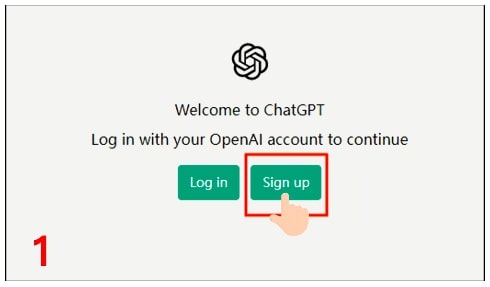
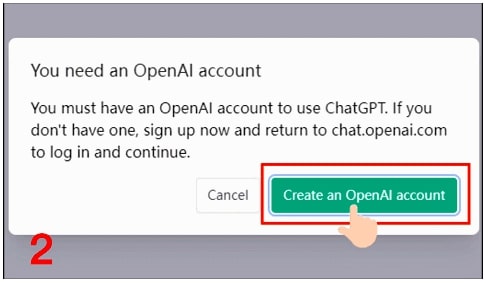

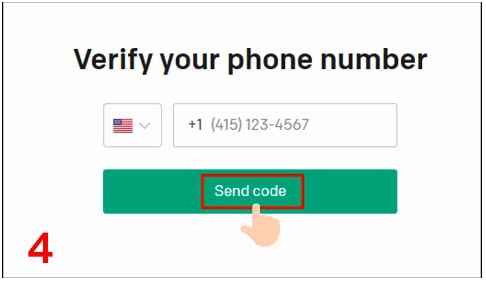


Functions of Chat GPT
ChatGPT is a type of language model that can be used for a variety of natural language processing tasks, such as:
Text Generation
Text Generation is a form of Artificial Intelligence (AI) that enables machines to generate text based on given data. This technology is used to produce natural language texts such as news articles, blog posts, and other content.
It can also be used to generate automated responses for customer service inquiries and create personalized content for marketing campaigns. Text generation has become increasingly popular as it allows businesses to save time and resources while still providing high-quality content.
ChatGPT generates text based on the patterns it learned during training. During training, the model is exposed to a large dataset of text, and it learns to identify patterns and relationships between words and phrases.
When it generates text, it uses these patterns to create new text that is similar to the input it was trained on, but not necessarily identical. This is why the generated text is not considered as plagiarism.
However, it’s important to note that there are ways to fine-tune the model to generate plagiarism-free content.
- One way is to train the model on a dataset that has been pre-processed to remove plagiarized text.
- Additionally, during the fine-tuning process, the model can be trained to recognize and avoid generating text that is identical or very similar to existing text.
- Another way is to use the API provided by OpenAI to generate text, they have a system that detects and filter out text that is too similar to existing text.
It’s also important to note that as a user, you should always verify the content generated by ChatGPT against existing text to ensure that it is not plagiarized.
Language Translation
Language translation is an important part of Artificial Intelligence (AI). AI tools are being used to translate text from one language to another, allowing companies and organizations to communicate with customers, partners, and colleagues in different countries.
AI-enabled language translation tools can help reduce the time and cost associated with translating documents or websites into multiple languages.
ChatGPT can be fine-tuned to perform language translation by training it on a dataset of parallel text in the source and target languages. This dataset is used to train the model to learn the relationship between the source and target languages, and to generate translations that are grammatically correct and semantically equivalent.
For example, you could fine-tune a pre-trained ChatGPT model on a dataset of English-French parallel text, and it would be able to translate English text into French.
The process of fine-tuning ChatGPT for language translation typically involves training the model on a large dataset of parallel text and adjusting the model’s hyperparameters to optimize its performance on the translation task.
Once the fine-tuning is done, you can use the model to translate text by providing it with an input sentence in the source language and it will generate the corresponding translation in the target language.
It’s important to note that ChatGPT is not a dedicated machine translation model and it is not as effective as state-of-the-art machine translation models. However, it can still provide decent translations and it is widely used in practice due to its flexibility and low cost.
Text Summarization
Text summarization is a process of reducing the length of a text document while preserving its key information. It is an important part of natural language processing (NLP) and artificial intelligence (AI).
By using text summarization, AI tools can quickly generate summaries from large documents, making them easier to read and understand.
ChatGPT can be fine-tuned to perform text summarization by training it on a dataset of text and corresponding summaries. This dataset is used to train the model to learn how to generate summaries that are coherent, concise, and semantically equivalent to the original text.
For example, you could fine-tune a pre-trained ChatGPT model on a dataset of news articles and their corresponding summaries, and it would be able to generate summaries of new articles.
The process of fine-tuning ChatGPT for text summarization typically involves training the model on a large dataset of text and summaries and adjusting the model’s hyperparameters to optimize its performance on the summarization task.
Once the fine-tuning is done, you can use the model to summarize text by providing it with an input text, and it will generate a summary.
There are different ways to train a GPT-based summarization model.
- One way is to train it as a sequence-to-sequence model where the input is the text to summarize and the output is the summary.
- Another way is to use a pointer-generator mechanism where the model can either generate a new summary or copy a part of the input text.
It’s important to note that summarization is a challenging task and the quality of the summaries generated by ChatGPT may vary depending on the quality and size of the training dataset, and the specific fine-tuning approach used.
Question Answering
Question Answering (QA) is an AI-based technology that enables machines to answer questions posed in natural language. It is a form of Natural Language Processing (NLP) that helps machines understand and respond to human queries. QA systems are used to provide answers to specific questions by finding relevant information from a large set of data such as documents, webpages, databases, etc.
ChatGPT can answer questions by using a technique called information retrieval. When a question is provided as input, the model retrieves relevant information from the text it was trained on, and generates an answer based on that information.
In order to answer questions in a few seconds, ChatGPT uses a pre-trained version of itself, that is fine-tuned on a large corpus of text that contains a vast amount of information on various topics, and it has been trained to identify patterns and relationships between words and phrases.
By having a pre-trained model, it doesn’t require a lot of computational power to generate an answer, it can quickly retrieve the information it needs from its memory.
Additionally, the model can be fine-tuned on a specific dataset that contains information relevant to a particular domain or topic, making it more efficient at answering questions within that domain.
It’s important to note that the quality and accuracy of the answers generated by ChatGPT will depend on the quality and coverage of the training data, and the complexity of the question.
Additionally, ChatGPT is a statistical model, so it may not always generate a correct answer and it’s not capable of understanding the meaning of the question, it just returns the most likely answer based on the patterns it learned from the training data.
Text classification
Text classification is a process of classifying text into different categories or classes. It is an important technique used in Artificial Intelligence (AI) tools to help machines understand the content of a text and classify it into predefined categories. Text classification helps AI tools to better analyze and interpret data, making them more accurate and efficient.
Text classification can be used for a variety of tasks such as sentiment analysis, topic modeling, spam filtering and document categorization. It can also be used to identify the topics or themes present in a given piece of text.
AI tools use text classification algorithms to accurately classify the text into different categories or classes. This helps them make decisions based on the classified information, making them more intelligent and efficient.
ChatGPT can perform text classification quickly by using a pre-trained version of itself, which has already learned patterns and relationships between words and phrases from a large dataset of text. This pre-trained model can be fine-tuned on a smaller dataset of labeled text for the specific text classification task.
The fine-tuning process typically involves adjusting the model’s hyperparameters and training it on a labeled dataset of text, where each text is assigned a specific category or label. The model learns to identify patterns and features in the text that are associated with specific categories, and uses these patterns to classify new text.
Once the fine-tuning is done, you can use the model to classify text by providing it with an input text, and it will predict the category or label that the text belongs to.
One way to fine-tune GPT-based model is to use a technique called “few-shot learning” where only a small number of labeled examples are used to fine-tune the model, this allows the model to be fine-tuned on specific tasks with very small datasets.
It’s important to note that the accuracy of the text classification task will depend on the quality and size of the training dataset, and the specific fine-tuning approach used. Additionally, the pre-trained GPT model was trained on a diverse set of texts, so if the text classification task is very specific and the texts are different from the training data, the model might not perform well.
Named Entity Recognition (NER)
Named entity recognition (NER) is a natural language processing task that involves identifying and classifying named entities in text, such as people, places, and organizations.
ChatGPT can perform named entity recognition by fine-tuning a pre-trained version of itself on a labeled dataset of text where named entities are annotated with specific tags or labels.
The fine-tuning process typically involves adjusting the model’s hyperparameters and training it on a labeled dataset of text, where each named entity is assigned a specific category or label.
The model learns to identify patterns and features in the text that are associated with specific named entities, and uses these patterns to classify new text.
Once the fine-tuning is done, you can use the model to classify named entities in text by providing it with an input text, and it will predict the named entities and the category or label that they belong to.
It’s important to note that the accuracy of the named entity recognition task will depend on the quality and size of the training dataset, and the specific fine-tuning approach used.
Additionally, the pre-trained GPT model was trained on a diverse set of texts, so if the named entity recognition task is very specific and the texts are different from the training data, the model might not perform well.
Also, Named Entity recognition is a complex task and GPT-based models are not as accurate as state-of-the-art NER models but they are still widely used due to their flexibility and low cost.
Sentiment analysis
Sentiment analysis, also known as opinion mining, is a natural language processing task that involves determining the sentiment or emotion expressed in text, such as whether it is positive, negative, or neutral.
ChatGPT can perform sentiment analysis by fine-tuning a pre-trained version of itself on a labeled dataset of text where the sentiment of the text is annotated with specific labels (e.g. positive, negative, neutral).
The fine-tuning process typically involves adjusting the model’s hyperparameters and training it on a labeled dataset of text, where each text is assigned a specific sentiment label.
The model learns to identify patterns and features in the text that are associated with specific sentiments, and uses these patterns to classify new text.
Once the fine-tuning is done, you can use the model to classify the sentiment of text by providing it with an input text, and it will predict the sentiment label that the text belongs to.
It’s important to note that the accuracy of the sentiment analysis task will depend on the quality and size of the training dataset, and the specific fine-tuning approach used.
Additionally, sentiment analysis is a complex task and GPT-based models are not as accurate as state-of-the-art sentiment analysis models but they are still widely used due to their flexibility and low cost.
Also, it is important to note that sentiment analysis is a challenging task and it is not only limited to determining whether a text is positive or negative, it also involves understanding the nuances and subtleties of the text and identify the sentiment expressed in a more fine-grained way.
Dialog systems
A dialog system, also known as a conversational agent or chatbot, is a computer program that can simulate conversation with human users through natural language processing and generation.
ChatGPT can be used to build dialog systems by fine-tuning a pre-trained version of itself on a dataset of conversational text.
The fine-tuning process typically involves adjusting the model’s hyperparameters and training it on a dataset of dialogs, where each dialog is a series of turns of conversation between a user and the system.
The model learns to identify patterns and features in the text that are associated with specific conversational intents and generate coherent and contextually appropriate responses.
Once the fine-tuning is done, you can use the model to generate responses to user inputs in a dialog. The model can be integrated into a dialog management system that handles the flow of the conversation, such as keeping track of the conversation context, handling user inputs and errors, and providing appropriate responses.
It’s important to note that building a dialog system is a complex task and requires not only a good understanding of natural language processing techniques but also knowledge about dialog management, user experience design, and other related areas.
Additionally, GPT-based models have shown to be good at generating human-like responses but they still have limitations when it comes to understanding the context and the user intent, which are critical for building a good dialog system.
Language modeling
Language modeling in AI involves training a model to predict the likelihood of a sequence of words. The model is trained on a large dataset of text, and the goal is for the model to generate text that is similar to the training data.
ChatGPT is a large language model that uses a variant of the transformer architecture to perform language modeling. It is trained on a massive dataset of text and can generate human-like responses to prompts.
The model is fine-tuned for various specific task such as question answering, text summarization, and dialog generation. When given a prompt, ChatGPT uses its trained knowledge of the patterns and structures of language to generate a response that is relevant and coherent.
Text completion
Text completion in AI is a task where the model is given a partially written sentence or phrase and is asked to generate the next word or set of words that complete the sentence. This task is also known as text generation or language generation.
ChatGPT is trained to perform text completion by being exposed to a large amount of text data. It learns the patterns, structure and grammar of the language by analyzing that data.
The model uses a technique called “autoregression” where the model is trained to predict the next word in a sequence given the previous words in that sequence.
When given a partially written sentence as a prompt, ChatGPT uses this trained knowledge to generate a continuation of the sentence that is contextually relevant and grammatically correct.
Text generation from prompts
Text generation from prompts in AI is a task where a model is given a prompt or a starting point, and it generates a sequence of text based on that prompt.
This task is also known as text completion or language generation. The prompt could be a starting sentence, a topic, or a question, and the generated text should be relevant to the prompt and coherent.
ChatGPT is an open AI model that is trained to perform text generation from prompts by being exposed to a large dataset of text. It learns the patterns, structure, and grammar of the language by analyzing that data.
When given a prompt, ChatGPT uses its trained knowledge of the patterns and structures of language to generate text that is relevant to the prompt and coherent.
It uses a variant of the transformer architecture which is trained on the massive datasets, and it uses the autoregression technique similar to text completion, where the model is trained to predict the next word in a sequence given the previous words in that sequence.
This allows the model to generate text that is contextually relevant and grammatically correct.
Text-to-Speech (TTS)
Text-to-speech (TTS) in AI is the process of converting written text into spoken words. This technology is used in a wide range of applications, such as virtual assistants, automated customer service systems, and assistive technology for people with visual impairments.
ChatGPT is a sophisticated AI Tool that is trained to perform text generation and text completion tasks, it is not specifically designed for TTS, it can be used to generate text which can be used as input for TTS models. The generated text is contextually relevant, grammatically correct and human-like.
- To perform TTS tasks quickly, one approach is to use a pre-trained TTS model, which can convert the text generated by ChatGPT into speech in real-time. These models are trained on large dataset of audio and text data and can generate speech that sounds natural and human-like.
- Another approach is to use a neural TTS model such as Tacotron 2 or WaveRNN, which can convert text into speech in real time. These models are trained on large datasets of audio and text data and can generate speech that sounds natural and human-like.
Automatic Speech Recognition (ASR)
Automatic Speech Recognition (ASR) is a technology that allows computers to convert spoken words into written text. This technology is used in a wide range of applications such as voice commands for smartphones, virtual assistants, and dictation software.
ChatGPT is a modern AI tool that is trained to perform language modeling, text completion and text generation tasks, it is not specifically designed for Automatic Speech Recognition. However, it can be used as a backend for an ASR system, where the model can be fine-tuned on a smaller dataset of audio and text data for a specific use-case and task.
- To perform Automatic Speech Recognition, one approach is to use pre-trained ASR models such as Deep Speech 2 or Kaldi. These models are trained on large datasets of audio and text data and can transcribe speech with high accuracy. They use deep learning techniques to learn the patterns in speech and convert it into text.
- Another approach is to use end-to-end ASR models, which can transcribe speech directly without the need for a separate language model. These models are trained on large datasets of audio and text data and can transcribe speech with high accuracy.
Text-to-Code
Text-to-Code in AI is the task of automatically converting natural language instructions into code. This technology is used in a wide range of applications such as code generation, programming by voice, and automating repetitive coding tasks.
ChatGPT is a modern AI software that is trained to perform language modeling, text generation, and text completion tasks, it is not specifically designed for Text-to-Code task. However, it can be used as a backend for a Text-to-Code system, where the model can be fine-tuned on a smaller dataset of code and natural language instructions for a specific use-case and task.
- To perform Text-to-Code task, one approach is to use pre-trained Text-to-Code models such as Code-BERT, which is a transformer-based model pre-trained on a massive amount of code and natural language instruction pairs. This model can generate code snippets, given natural language instructions or complete a partially written code.
- Another approach is to use end-to-end Text-to-Code models, which can generate code snippets directly from natural language instructions without the need for a separate language model. These models are trained on large datasets of code and natural language instructions and can generate code with high accuracy and readability.
It’s worth to mention that Text-to-Code models are still in a research phase, and the generated code may not always run correctly or be of production-level quality.
Poem and Song Lyrics Generation
Poem and Song Lyrics Generation in AI is the task of automatically generating creative text such as poetry or song lyrics. This technology can be used to generate new works of poetry or song lyrics, or to assist writers and musicians with the creative process.
ChatGPT is an advanced AI tool that is trained to perform language modeling, text generation, and text completion tasks. It can be fine-tuned on a smaller dataset of poetry or song lyrics to generate new works of poetry or song lyrics.
- To perform Poem and Song Lyrics Generation task, one approach is to use pre-trained models such as GPT-3. These models are fine-tuned on a dataset of poetry or song lyrics and can generate new works that are contextually relevant, grammatically correct, and have a similar style to the training data.
- Another approach is to use a rule-based approach where a set of predefined rules are used to generate poem or song lyrics based on certain parameters such as rhyme, meter, and structure.
It’s worth to mention that Poem and Song Lyrics Generation is a challenging task that requires a good understanding of poetry and songwriting, and the results may not be as good as those written by a human. Additionally, the generated text may not have the same emotional depth and creativity as those written by a human.
Text-to-Emoji
Text-to-emoji in AI is the task of automatically converting natural language text into emojis, which are small graphical images or pictograms that are used to express emotions or ideas. This technology is used in a wide range of applications such as social media, instant messaging, and chatbots.
ChatGPT is a famous AI software that is trained to perform language modeling, text generation, and text completion tasks, it is not specifically designed for Text-to-emoji task. However, it can be used as a backend for a Text-to-emoji system, where the model can be fine-tuned on a smaller dataset of natural language text and corresponding emojis for a specific use-case and task.
- To perform Text-to-emoji task, one approach is to use pre-trained Text-to-emoji models such as EmojiNet, which is a model pre-trained on a massive amount of text and emojis pairs. This model can generate emojis, given natural language text.
- Another approach is to use a rule-based approach where a set of predefined rules are used to map certain words or phrases to specific emojis. This approach can be less accurate than machine learning models but it can be faster and simpler to implement.
It’s worth to mention that Text-to-emoji is still a relatively new area of research, and the results may not be as accurate or comprehensive as other AI tasks.
Text-to-Images
Text-to-images function in AI is the task of automatically generating images from natural language descriptions. This technology is used in a wide range of applications such as image generation, computer vision, and virtual reality.
ChatGPT is a large language model that is trained to perform language modeling, text generation, and text completion tasks. It is not specifically designed for Text-to-images tasks.
However, it can be used as a backend for a Text-to-images system, where the model can be fine-tuned on a smaller dataset of natural language descriptions and corresponding images for a specific use-case and task.
- To perform the Text-to-images tasks, one approach is to use pre-trained Text-to-images models such as DALL-E, which is a transformer-based model pre-trained on a massive amount of text and image pairs. This model can generate images, given natural language descriptions.
- Another approach is to use a Generative Adversarial Network (GAN) model, which is trained to generate new images that look similar to the training images, given a textual description or a set of attributes.
It’s worth mentioning that Text-to-images is a challenging task that requires high computational power and large datasets to train, and the results may not be as accurate or realistic as other AI tasks.
Text-to-gifs
A gif (short for Graphics Interchange Format) is a type of image file that can be used to create simple animations or short video clips. They are often used in social media, instant messaging, and online forums to convey emotions or ideas in a more engaging and visual way.
ChatGPT is a renowned AI software that is trained to perform language modeling, text generation, and text completion tasks. It is not specifically designed for Text-to-gifs task. However, it can be used as a backend for a Text-to-gifs system, where the model can be fine-tuned on a smaller dataset of natural language descriptions and corresponding gifs for a specific use-case and task.
- To perform Text-to-gifs task, one approach is to use pre-trained Text-to-gifs models such as GPT-3-SVG, which is a transformer-based model pre-trained on a massive amount of text and gifs pairs. This model can generate gifs, given natural language descriptions.
- Another approach is to use a Generative Adversarial Network (GAN) model, which is trained to generate new gifs that look similar to the training gifs, given a textual description or a set of attributes.
It’s worth to mention that Text-to-gifs is a challenging task that requires high computational power and large datasets to train, and the results may not be as accurate or realistic as other AI tasks. Additionally, it might not be able to generate gifs in real-time, but it can be used to generate a list of gifs that can be used for a specific task or context.
Text-to-Questions
Text-to-Questions in AI is the task of automatically generating questions from a given text. This technology is used in a wide range of applications such as question-answering, educational technology, and information retrieval.
ChatGPT is a modern and sophisticated tool that is trained to perform language modeling, text generation, and text completion tasks. It can be fine-tuned on a smaller dataset of text and corresponding questions to handle Text-to-Questions tasks.
- To perform Text-to-Questions task, one approach is to use pre-trained models such as GPT-3, which is fine-tuned on a dataset of text and corresponding questions. This model can generate questions that are contextually relevant and grammatically correct, given a piece of text.
- Another approach is to use a rule-based approach where a set of predefined rules are used to generate questions based on the structure of the text, the type of text, or the desired type of question.
It’s worth mentioning that Text-to-Questions is a challenging task that requires a good understanding of natural language processing and question-answering, and the results may not be as accurate or comprehensive as other AI tasks. Additionally, the generated questions may not always be meaningful, relevant or useful for the given text.
Coding and Programming Via Chat GPT
Programming and Coding Generation via AI is the task of automatically generating computer and web programming code from natural language descriptions. This technology is used in a wide range of applications such as code generation, programming by voice, and automating repetitive coding tasks.
ChatGPT is a modern AI software that is trained to perform language modeling, text generation, and text completion tasks. It is not specifically designed for Programming and Coding Generation tasks.
However, it can be used as a backend for a Programming and Coding Generation system, where the model can be fine-tuned on a smaller dataset of natural language descriptions and corresponding code for a specific use case and task.
- To perform the Programming and Coding Generation task, one approach is to use pre-trained Programming and Coding Generation models such as Code-BERT, which is a transformer-based model pre-trained on a massive amount of code and natural language instructions pairs. This model can generate code snippets, give natural language instructions, or complete a partially written code.
- Another approach is to use end-to-end Programming and Coding Generation models, which can generate code snippets directly from natural language instructions without the need for a separate language model. These models are trained on large datasets of code and natural language instructions and can generate code with high accuracy and readability.
It’s worth mentioning that Programming and Coding Generation is a challenging task that requires high computational power and large datasets to train, and the generated code may not always run correctly or be of production-level quality.
Limitations of Chat GPT
AI content-generating tools are a great way to produce content quickly and efficiently. However, there are some potential limitations and drawbacks that should be considered when using these tools.
Lack of commonsense reasoning: ChatGPT, like other language models, lacks the ability to understand and reason about the world in the same way that humans do. This can lead to nonsensical or irrelevant responses when the model is prompted with certain types of questions or tasks.
Limited understanding of context: It does not have the ability to understand the context in which the text was written. This can lead to confusion or misinterpretation when the model is prompted with text that has a different meaning in a different context.
Limited knowledge of the world: It does not have a comprehensive understanding of the world. This can lead to errors or inaccuracies when the model is prompted with questions about facts or events.
Bias: This tool is trained on a large dataset of text, which can include biases present in the data. This can lead to the model perpetuating these biases in its responses.
Limited ability to understand idioms and sarcasm: It may not be able to fully understand idioms or sarcasm. This can lead to confusion or misinterpretation in the model’s responses.
Difficulty in handling tasks that require a lot of background knowledge: ChatGPT may not have the necessary background knowledge to handle certain tasks.
Limited ability to understand and generate emotions: ChatGPT may not have the ability to understand and generate emotions in the same way that humans do.
Limited ability to understand and generate Images, Gifs, and Video: ChatGPT is a language model and it’s not specifically designed to understand and generate images, gifs, and videos.
Limited ability to understand and generate Audio: ChatGPT is a language model and it’s not specifically designed to understand and generate audio.
Limited ability to understand and generate code: ChatGPT is not specifically designed to understand and generate code, it can generate code snippets, but the generated code may not always run correctly or be of production-level quality.
Chat GPT and Content Creation
In the modern era, content is king, and creating content is the best way to reach out to your target audience. Content creation is an essential part of marketing and branding strategies. It helps businesses to build a strong presence on the internet, boost their visibility, attract more customers, and increase their sales.
Chat GPT has revolutionized content creation as below:
Increased speed and efficiency: ChatGPT can generate text quickly and efficiently, which can save time and resources for content creators.
Improved personalization: It can generate text that is personalized to a specific audience or use case, which can improve engagement and relevance for the reader.
Increased creativity: This tool can generate text that is creative and original, which can help content creators to come up with new and unique ideas.
Improved language understanding: ChatGPT has a deep understanding of language, which can help content creators to improve the quality of their text.
Improved accessibility: It can generate text in multiple languages, which can help to make content more accessible for a global audience.
Improved SEO: ChatGPT can generate text that is optimized for search engines, which can help to improve the visibility and reach of content.
Improved consistency: This tool can generate text that is consistent in tone, style, and voice, which can help to improve the overall quality of content.
Improved scalability: This advanced AI software can generate large amounts of text quickly and efficiently, which can help to scale content creation efforts.
Improved efficiency in repetitive tasks: ChatGPT can be fine-tuned on a smaller dataset of text to generate repetitive tasks such as summaries, captions.
Chat GPT and Content Marketing
Chat GPT, a state-of-the-art language model, can play a significant role in modern content marketing strategies. Here are a few ways in which Chat GPT can be used to improve content marketing efforts:
Content generation: Chat GPT can generate high-quality, engaging text that can be used to create blog posts, articles, social media posts, and other types of content.
Personalization: Chat GPT can generate text that is personalized to a specific audience or use case, which can improve engagement and relevance for the reader.
SEO optimization: Chat GPT can generate text that is optimized for search engines, which can help to improve the visibility and reach of content.
Content summarization: Chat GPT can summarize large bodies of text, which can be useful in creating summaries of long-form content such as research reports or whitepapers.
Content localization: Chat GPT can generate text in multiple languages, which can help to make content more accessible to a global audience. It would become very helpful in Local SEO.
Content curation: Chat GPT can be fine-tuned on a smaller dataset of text to generate text that curates or summarize a specific subject, and this can be useful in creating curated content such as newsletter, news brief, and more
Social Media: Chat GPT can be fine-tuned on a smaller dataset of text to generate text that is suitable for social media platforms such as captions, hashtags, and more.
Chatbot interactions: Chat GPT can be used to improve the conversational capabilities of chatbots, which can be used in customer service and other interactive marketing efforts.
Email Marketing: Chat GPT can be fine-tuned on a smaller dataset of text to generate text that is suitable for email marketing, such as subject lines, body content, and more.
Overall, Chat GPT can be a powerful tool for content marketers, as it can help to improve the quality, relevance, and reach of content, while also saving time and resources.
Checklist Before Posting Chat GPT Content Online
Before posting AI-generated content online, it is important to ensure that the content is accurate and up-to-date. It should also be checked for any potential errors or inconsistencies.
Additionally, the content should be checked for plagiarism and copyright infringements. Furthermore, it is important to make sure that the content meets the requirements of search engine optimization (SEO) and other online marketing techniques so that it can reach its intended audience.
Finally, it is important to check if the AI-generated content follows ethical standards and regulations in order to protect users’ privacy.
Let me give you a checklist, which we should follow before copy-pasting AI-generating content online:
Grammar and spelling: Before posting content generated by Chat GPT online, it’s important to check that the grammar and spelling are correct.
Relevance: Ensure that the content generated by Chat GPT is relevant to the intended audience and the purpose of the post.
Fact-checking: Verify any information or statistics included in the content to ensure that they are accurate and up-to-date.
Bias: Check for any biases present in the content and consider if it might offend or alienate any audience members.
Quality: Ensure that the content is of high-quality, well-written and engaging.
Branding and Tone: Check if the content aligns with the company’s brand and tone of voice, if it’s necessary.
Compliance: Ensure that the content adheres to any legal or compliance regulations and guidelines.
Optimization: Optimize the content for search engines if it’s intended for the web.
Formatting: Check if the content is formatted correctly for the intended platform.
User experience: Review the content from a user’s perspective and ensure that it is easy to read and understand.
Though creating and posting AI-generated content is not against Google’s policy, however, as per Google Quality Raters Guidelines, the tech giant always prefers to EAT content in YMYL topics, therefore, it is mandatory to re-check the quality and authenticity of the content before posting online.
Comparison of Content Generating AI Tools
Here is the comparison between Chat GPT and other widely known language models such as GPT-3 and BERT. Here is a table that illustrates the main differences between these models:
Chat GPT | GPT-3 | BERT | |
---|---|---|---|
Model architecture | Transformer | Transformer | Transformer |
Training dataset | Common Crawl | Common Crawl | Wikipedia + BooksCorpus |
Fine-tuning capabilities | Yes | Yes | Yes |
Pre-training task | Language Modeling | Language Modeling | Masked Language Modeling |
Pre-training data size | 10 terabytes | 570 GB | 340 GB |
Pre-training time | Not specified | Not specified | 4 days |
Number of parameters | 1.5 billion | 175 billion | 110 million |
Fine-tuning data size | Not specified | Not specified | Not specified |
Fine-tuning time | Not specified | Not specified | Not specified |
Availability | Open-source | API service (paid) | Open-source |
Please note that this table only includes general information and the values might not be accurate or up-to-date.
What is Chat GPT Professional?

ChatGPT Professional is a premium version of OpenAI’s popular ChatGPT chatbot. It will be introduced as a pilot program in 2023, with the aim of providing businesses and organizations with access to a more advanced version of the chatbot for commercial use.
The premium version of ChatGPT includes a number of additional features and capabilities over the standard version, including:
- Increased capacity and performance, allowing the chatbot to handle more complex tasks and handle more users at once.
- Improved customization options, allowing businesses to fine-tune the chatbot to better suit their specific needs and use cases.
- Access to a dedicated support team and additional resources for training and fine-tuning the chatbot.
The pilot program for ChatGPT Professional was aimed at gathering feedback from businesses and organizations to improve the model and its features.
The aim is to make the model more efficient, easier to use, and adaptable to a wide range of use cases to help businesses automate some of their processes, improve customer service and generate new revenue streams.
Bottom Line
ChatGPT is a type of language model that can be used for a variety of natural language processing tasks, such as:
- Text generation: ChatGPT can generate text that is similar to the input it was trained on.
- Language translation: It can be fine-tuned to translate text from one language to another.
- Text summarization: ChatGPT can generate a summary of a given text.
- Question answering: It can generate answers to questions based on a given context.
- Text classification: ChatGPT can classify text into different categories.
- Named entity recognition: It can identify and classify named entities in text, such as people, places, and organizations.
- Sentiment analysis: ChatGPT can determine the sentiment of text, such as whether it is positive, negative, or neutral.
- Dialog systems: It can be used to build conversational agents and chatbots.
- Language modeling: ChatGPT can be used to predict the next word in a sentence or generate text that is grammatically correct.
- Text completion: It can complete a partially written sentence or passage.
- Text generation from prompts: ChatGPT can generate text based on a given prompt or topic.
- Text-to-speech: It can be used to generate speech from text.
- Automatic speech recognition: ChatGPT can be fine-tuned to transcribe spoken language into text.
- Text-to-code: It can be used to generate code snippets from natural language
- Poem and song lyrics generation
- Text-to-emoji: ChatGPT can be used to generate emojis that best represents the input text
- Text-to-images: It can be used to generate images based on text descriptions
- Text-to-gifs: ChatGPT can be used to generate gifs based on text descriptions
- Text-to-questions: It can be used to generate questions based on a given text
- Text-to-commands: ChatGPT can be used to generate commands based on a given text
ChatGPT uses a transformer architecture, which allows it to process input text of any length and generate output text that is coherent and contextually appropriate.
It uses a technique called unsupervised pre-training, which means that the model is trained on a massive dataset of unlabelled text before being fine-tuned for specific tasks.
Sharing is Caring